Enterprise Risk Management Formula Book
5. Statistical Methods
[this page | pdf | back links]
5.1 Sample moments
A random sample of
observations
has (equally
weighted) sample moments as follows:
‘Population’ moments (e.g. population variance,
population skewness,
population excess
kurtosis) are calculated as if the distribution from which the data was
being drawn was discrete and the probabilities of occurrence exactly matched
the observed frequency of occurrence.
The least squares estimator for parameters of a distribution
are the values of the parameters that minimise the square of the residuals, so
the least squares estimator for the mean,
, is the value
that minimises 
Non-equally weighted moments give different weights to
different observations (the weights not dependent on the ordering of the
observations), e.g. the sample non-equally weighted mean (using
weights
) is:

5.2 Parametric inference (with an underlying
following the normal distribution)
One sample:
For a single (equally weighted) sample of size
,
, where
then the
following statistics are distributed according to the Student’s t
distribution and the chi-squared distribution:

Two samples:
For two independent samples of sizes
and
,
and
, where
and
then the
following statistic is
distributed according to the F distribution:
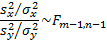
If
then:
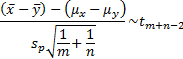
where
is the pooled
sample variance.
5.3 Maximum likelihood estimators
If
is the maximum likelihood estimator
of a parameter
based on a
sample
then

where
is the
likelihood for the sample, i.e.
and hence 
is
asymptotically normally distributed with mean
and
variance equal to the Cramér-Rao lower bound
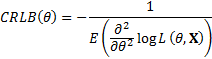
Likelihood ratio test:
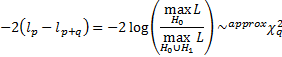
where
is the
maximum log-likelihood for the model under
(with
free
parameters) and
is the
maximum log-likelihood for the model under
(with
free
parameters). Non-equally weighted estimators can be identified by weighting the
terms
appropriately.
5.4 Method-of-moments estimators
Method of moments estimators are the parameter values
(for the
parameters
specifying a given distributional family) that result in replication of the
first
moments of
the observed data. For the normal distribution these involve
and either
(the sample
variance, if a small sample size adjustment is included) or
(the
‘population’ variance, if the small sample size adjustment is ignored and we
select the estimators to fit
and
. In the generalised
method of moments approach we select parameters that ‘best’ fit the
selected moments (given some criterion for ‘best’), rather than selecting
parameters that perfectly fit the selected moments.
5.5 Goodness of fit
Goodness of fit describes how well a statistical model fits
a set of observations. Examples include the following, where
is the
’th
order statistic,
is the
supremum (i.e. largest value) of the set
,
is
the cumulative distribution function of the distribution we are fitting and
is the
empirical distribution function:
(a) Kolmogorov-Smirnov
test:
. Under the
null hypothesis (that the sample comes from the hypothesized distribution), as
then
tends to a
limiting distribution (the Kolmogorov distribution).
(b) Cramér-von-Mises test: 
(c) Anderson-Darling test:
where 
If data is bucketed into ranges then we may also use
(Pearson’s) chi-squared goodness of fit test using the following test
statistic, where
is the sample
size and
is the
observed count,
is the
expected count and
and
are the lower
and upper limits for the
’th bin. The test
statistic follows approximately a chi-squared distribution with
degrees
of freedom, i.e.
where
is
the number of non-empty cells and
is the number
of estimated parameters plus 1:
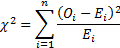
We may also test whether the skew or kurtosis or the two
combined (the Jarque-Bera test) appear materially different from what would be
implied by the relevant distributional family. If the null hypothesis is that
the data comes from a normal distribution then, for large
,
,
and
.
The Akaike
Information Criterion (AIC) (and other similar ways of choosing between
different types of model that trade-off goodness of fit with model complexity,
such as the Bayes Information Criterion, BIC) involves selecting the
model with the highest information criterion of the form
where there
are
unknown
parameters and we are using a data series of length
for
fitting purposes. For the AIC
and for the
BIC
.
5.6 Linear regression
In the univariate case suppose
where
,
then
(equally weighted) estimates of
and
are:


where
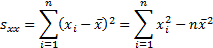
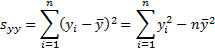

Also

The individual expected responses are
and satisfy
the following ‘sum of squares’ relationship:

The variance of the predicted mean response is:

The variance of a predicted individual response is the
variance of the predicted mean response plus an additional
.
For generalised least squares, if we have
different
series each with
observations
we are fitting
then the
vector of least squares estimators,
is given by
where
is a
matrix
with elements
and
is an
dimensional
vector with elements
.
5.7 Correlations
The observed (sample) correlation coefficient
(i.e. Pearson correlation coefficient) between two series of equal
lengths indexed in the same manner
and
is (where
,
and
are as given
in the section on linear regression):

If the underlying correlation coefficient,
,
is zero and the data comes from a bivariate normal distribution then:

For arbitrary
(
)
the Fisher z transform
is
where:

If the data comes from a bivariate normal distribution then
is
distributed approximately as follows:

Two non-parametric measures of correlation are:
-
Spearman’s
rank correlation coefficient, where
and
are the ranks
within
and
of
and
respectively:

-
Kendall’s
tau, where computation is taken over all
and
with
and
(for the moment ignoring ties) a concordant pair is a case where
and a
discordant pair is a case where
:

There are various possible ways of handling ties in these
two non-parametric measures of correlation (ties should not in practice arise
if the random variables really are continuous).
5.8 Analysis of variance
Given a single factor normal model

where
with
.
Variance estimate:

Under the null hypothesis given above
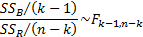
where:

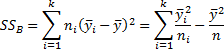

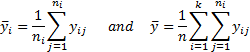
5.9 Bayesian priors and posteriors
Posterior and prior distributions are related as follows:

i.e.

For example, if
is a random
sample of size
from a
where
is known and
the prior distribution for
is
then the
posterior distribution for
is:

where
is
‘credibility weighted’ as follows:

and
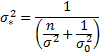
NAVIGATION LINKS
Contents | Prev | Next