Estimating operational risk capital
requirements assuming data follows a bi-uniform or a triangular distribution
(using maximum likelihood)
[this page | pdf | back links]
Suppose a risk manager believes that an appropriate model
for a particular type of operational risk exposure involves the loss,
,
never exceeding an upper limit,
,
and the probability density function
taking
the form of a bi-uniform distribution:
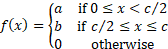
where
are
all constant.
Suppose we want to estimate maximum likelihood estimators
for
,
and
given
losses of
,
say and hence to estimate a Value-at-Risk for a given confidence level for this
loss type, assuming that the probability distribution has the form set out
above.
We note that
for
to
correspond to a probability density function, so:


Suppose the
losses,
,
are assumed to be independent draws from a distribution with probability
density function
and
suppose
of
these losses are less
and
are
greater than
.
The likelihood is then:

This will be maximised for some value that has
,
i.e. has
at
least as large as
.
In such circumstances the likelihood is maximised when the log likelihood is
maximised which will be when
,
i.e. when
,
i.e. when:
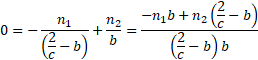

(assuming
and
)
For these values of
and
the
log likelihood is then:

In most circumstances this will be maximised when
is
as small as possible, provided
is
still at least as large as
so
the maximum likelihood estimators are:

However, it is occasionally necessary to consider the case
where we select a
and
have
and/or
equal
to zero.
To estimate a VaR at a confidence level
we
need to find the value
for
which the loss is expected to exceed
only
%
of the time, i.e.
such
that (if
):
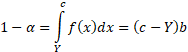
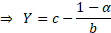
A similar approach can be used for tri-uniform distributions
or other more complex piecewise uniform distributions.
Somewhat more complex is if
takes
the form of a triangular distribution, i.e. where:
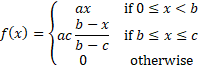
where
are
all constant.
Nguyen
and McLachlan (2016), when developing a new algorithm for maximum
likelihood estimation of triangular or more general polygonal distributions,
note that in the case where
and
then
Oliver (1972), “A maximum likelihood oddity”, American Statistician 26, 43–44, indicates
that
for
some
and
moreover that if the sample is ordered so that
then
where:

Asymptotically, on average, the number of observations in
appears
to be approximately two.
We can apply this reasoning to the more general triangular
distribution where we do not know
by
again noting that in many cases the log-likelihood will be minimised by setting
to
be as small as possible, provided
is
still at least as large as
,
and then separately handling cases where the likelihood can be improved by
selecting a higher value for
.