Derivation of the Cornish-Fisher
asymptotic expansion
[this page | pdf | references | back links]
A common methodology within risk management circles for
estimating the shape of a fat-tailed return distribution is to make use of the
Cornish-Fisher asymptotic expansion, see e.g. Abramowitz
and Stegun (1970). The Cornish Fisher asymptotic expansion in effect takes
into account non-Normality, and thus by implication moments higher than the
second moment, by using a formula in which terms in higher order moments
explicitly appear. Most commonly the focus is on the fourth-moment version of
this expansion, since it merely uses moments up to and including kurtosis. In
effect, the fourth-moment Cornish Fisher approach aims to provide a reliable
estimate of the distribution’s entire quantile-quantile plot merely from the
first four moments of the distribution, i.e. its mean, standard deviation, skew
and kurtosis.
The approach works as follows. Let
be identically
distributed random variables. Let the cumulative distribution function of
be denoted by
. Then the
(Cornish-Fisher) asymptotic expansion (with respect to
) for
the value of
such that
is
where:

Here terms in brackets are terms of the same order with
respect to
,
is
the mean of the distribution,
the standard deviation
of the distribution and
are the distribution’s
cumulants, i.e. the coefficients of the following power series expansion
for
where
is the distribution’s characteristic
function.

The cumulants are related to the moments of the distribution
via the relationship
(for
)
where
is the skew(ness),
is the (excess)
kurtosis etc.
is the relevant
cumulative Normal distribution point, i.e. the value for which:
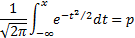
and

The
are the Hermite
polynomials, i.e.:
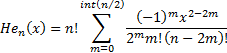
In effect, if we are using a fourth moment Cornish-Fisher
adjustment then this means estimating the shape of a quantile-quantile plot by
the following cubic, where
is the skew and
is the kurtosis of
the distribution:

For standardised returns (with
and
), this simplifies to:
