Enterprise Risk Management Formula Book
11. Extreme value theory
[this page | pdf | back links]
See also here.
11.1 Maximum domain of attraction (MDA)
Suppose that i.i.d. random variables
have cdf
. Suppose also
that there exist sequences
and
and a cdf
such that:

where
is the random
variable corresponding to the block maximum for blocks of such variables of
length
, i.e. each
(independent) realisation of the series
is used to
create a realisation of
given by
.
Then
is said to be
in the maximum domain of attraction (MDA) of
,
written 
11.2 Fisher-Tippett theorem
If
where
is
a non-degenerate cdf then
must be a
Generalised Extreme Value (GEV) distribution.
If
where
then by
replacing
by
and
by
we see that
where
.
11.3 The Pickands-Balkema-de Haan (PBH) theorem
Let
be the
maximum limiting value of the random variable
.
Then the PBH theorem states that we can find a function
such that

if and only if that 
11.4 Estimating tail distributions
Suppose that the underlying loss distribution is in the
maximum domain of attraction of the Frechét distribution and it has a tail of
the form:
for some
slowly varying
, where
. Then the
Hill estimator for the (upper) tail index, given
ordered
observations,
, assuming
that the (upper) tail contains
entries is:
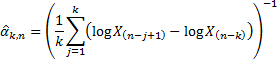
NAVIGATION LINKS
Contents | Prev | Next