Enterprise Risk Management Formula Book
Appendix A.2: Probability Distributions:
Continuous (univariate) distributions (a) Normal, uniform, chi-squared
[this page | pdf]
Distribution name
Normal distribution
Common notation

Parameters
= scale
parameter (
)
= location
parameter
Domain

Probability density
function

Cumulative distribution
function

Mean

Variance

Skewness

(Excess) kurtosis

Characteristic function

Other comments
The normal distribution is also called the Gaussian
distribution. The unit normal (or standard normal) distribution
is
.
The inverse unit normal distribution function (i.e. its
quantile function) is commonly written
(also in
some texts
and the
unit normal density function is commonly written
.
is also
called the probit function.
The error function distribution is
, where
is
now an inverse scale parameter
.
The median and mode of a normal distribution are
.
The truncated first moments of
are:

where
and
are the
pdf and cdf of the unit normal distribution respectively.
The mean excess function of a standard normal distribution
is thus

The central moments of the normal distribution are:

Distribution name
|
Uniform distribution
|
Common notation
|

|
Parameters
|
= boundary
parameters ( )
|
Domain
|

|
Probability density function
|

|
Cumulative distribution function
|

|
Mean
|

|
Variance
|

|
Skewness
|

|
(Excess) kurtosis
|

|
Characteristic function
|

|
Other comments
|
Its non-central moments ( are
. Its
median is .
|
Distribution name
|
Chi-squared
distribution
|
Common notation
|

|
Parameters
|
= degrees
of freedom (positive integer)
|
Domain
|

|
Probability density
function
|
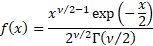
|
Cumulative distribution
function
|
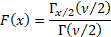
|
Mean
|

|
Variance
|

|
Skewness
|

|
(Excess) kurtosis
|

|
Characteristic function
|

|
Other comments
|
Its median is approximately . Its mode
is . Is also
known as the central chi-squared distribution (when there is a need to
contrast it with the noncentral chi-squared distribution).
In the special case of the
cumulative distribution function simplifies to .
The chi-squared distribution with degrees
of freedom is the distribution of a sum of the squares of independent
standard normal random variables. A consequence is that the sum of independent
chi-squared variables is also chi-squared distributed. It is widely used in
hypothesis testing, goodness of fit analysis or in constructing confidence
intervals. It is a special case of the gamma distribution.
As , and 
|
NAVIGATION LINKS
Contents | Prev | Next